[This blog has been dormant a long time, since I post most of this kind of content on Google+ these days. I’ll still cross-post the occasional longer piece here.]
There’s an important paper at ICML this week, showing results from a Google X project which scaled up deep learning to 16,000 cores. Just by throwing more computation at the problem, things moved substantially beyond the prior state of the art.
Building High-level Features Using Large Scale Unsupervised Learning
Quoc V. Le, Marc’Aurelio Ranzato, Rajat Monga, Matthieu Devin, Kai Chen, Greg S. Corrado, Jeffrey Dean, and Andrew Y. Ng
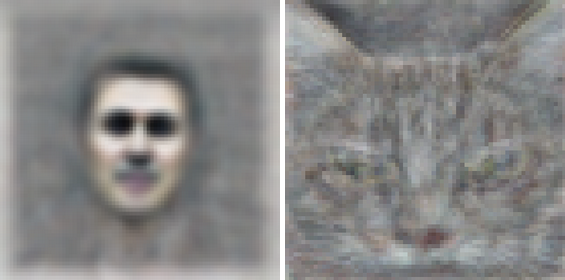
I think this is a really important paper, so let me give some background. Since starting at Google, there have been five projects which I’ve seen internally that blew me away with their obvious potential to truly change the world. A few of those are now public: (1) Self-driving cars, (2) Project Glass, (3) Knowledge Graph. Number four is this paper. It might grab fewer headlines than the first three, but in the long term this is by far the most important.
What this paper demonstrates (or at least convinced me) is that raw computation is now the key limiting factor for machine learning. That is a huge. For the last twenty years or more, it was not really the case. The field was dominated by SVMs and Boosting. Progress didn’t really have much to do with Moore’s Law. If machines got a million times faster, it wasn’t really clear that we had any good way to use the extra computation. There certainly wasn’t a viable path to animal-level perceptual abilities. Now I would like to stick my neck out and say that I think that position has changed. I think we now have a research program that has a meaningful chance of arriving at learning abilities comparable to biological systems.
That doesn’t mean that if someone gifted us a datacenter from 2050 we could solve machine learning immediately. There is a lot of algorithmic progress still to be made [1]. Unlike SVMs, the training of these systems still owes a lot to black magic. There are saturation issues that I think nobody has really figured out yet, to name one of a hundred problems [2]. But, the way seems navigable. I’ve been optimistic about this research ever since I saw Geoff Hinton’s talk on RBMs back in 2007, but it was a cautious optimism back then. Now that Google has shown you can scale the methods up by orders of magnitude and get corresponding performance improvements, my level of confidence has gone up several notches.
Returning to the present, here are a few cool aspects of the current paper:
1) Without supervision, the model learns complex, generalizable features (see the human face and cat face detectors below). To say that again, there is no labelled training data. Nobody told the model to detect faces. The face feature simply emerges naturally as a compact way for the network to reconstruct its inputs. We’ve seen that before for low level features like edges and edge junctions, but to see it for high level concepts is a result.
2) “Control experiments show that this feature detector is robust not only to translation but also to scaling and out-of-plane rotation.”
This is important too. It’s been known for a while that most current approaches used in computer vision don’t really learn any meaningful invariance to transformations which are not explicitly hand-designed into the features. e.g. See this paper from the DiCarlo lab: Comparing State-of-the-Art Visual Features on Invariant Object Recognition Tasks
3) “Starting with these learned features, we trained our network to obtain 15.8% accuracy in recognizing 20,000 object categories from ImageNet, a leap of 70% relative improvement over the previous state-of-the-art.”
It works! Well – it still fails 85% of the time (on a very very hard test set), but it’s big progress. These techniques apply to everything from speech recognition to language modeling. Exciting times.
——————————-
Notes:
[1]: I saw a talk by Geoff Hinton just yesterday which contained a big advance which he called “dropout”. No paper available yet, but check his website in the next few days. Lots happening right now.
[2] Or the embarrassing fact that models that achieve close to record performance on MNIST totally fail on 1 – MNIST (i.e. just invert the colours and the model fails to learn). Another example is the structural parameters (how many layers, how wide) which are still picked more or less arbitrarily. The brain is not an amorphous porridge, in some places the structure is important and the details will take years for us to figure out.